Sensing Noticeability in Ambient Information Environments
Yi Fei Cheng,
David Lindlbauer.
Published at
ACM CHI
2025
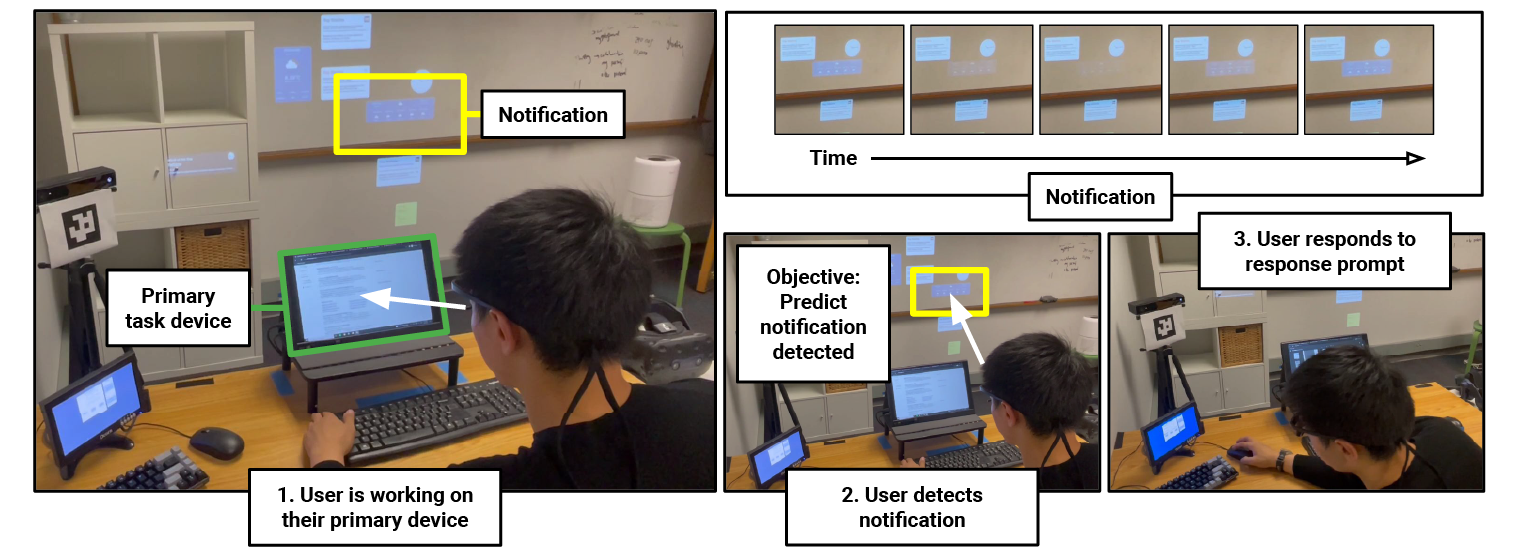
Abstract
Designing notifications in Augmented Reality (AR) that are noticeable yet unobtrusive is challenging since achieving this balance heavily depends on the user’s context. However, current AR systems tend to be context-agnostic and require explicit feedback to determine whether a user has noticed a notification. This limitation restricts AR systems from providing timely notifications that are integrated with users’ activities. To address this challenge, we studied how sensors can infer users’ detection of notifications while they work in an office setting. We collected 98 hours of data from 12 users, including their gaze, head position, computer interactions, and engagement levels. Our findings showed that combining gaze and engagement data most accurately classified noticeability (AUC = 0.81). Even without engagement data, the accuracy was still high (AUC = 0.76). Our study also examines time windowing methods and compares general and personalized models.
Materials
Bibtex
@inproceedings {Cheng25SensingNoticeability, author = {Cheng, Yi Fei and Lindlbauer, David}, title = {Sensing Noticeability in Ambient Information Environments}, year = {2025}, publisher = {Association for Computing Machinery}, address = {New York, NY, USA}, keywords = {Ambient displays, noticeability, computational interaction}, location = {Yokohama, Japan}, series = {CHI '25} }